XAIDA WEBINAR | Identifying probabilistic weather regimes targeted to a local-scale impact variable based on a modified variational autoencoder architecture
XAIDA is now hosting an open monthly webinar. Within the XAIDA project, sixteen research institutes and climate risk practitioners, aim to develop and apply novel artificial intelligence methods to better assess and predict the influence of climate change on extreme weather. Join the webinar each month to dive into interesting topics such as machine learning for climate extremes, the societal impact of extremes, and education about climate change.
Coordination: Maria Gonzalez-Calabuig (University of València), Manon Rousselle (IPSL)
November 5th at 2 PM (CET)
Speaker: Fiona Spuler, University of Reading (UR)
Title: Identifying probabilistic weather regimes targeted to a local-scale impact variable based on a modified variational autoencoder architecture
Abstract: Atmospheric circulation regimes act as mediators between long-range teleconnections and local impacts that have shown promise for improving predictions across timescales (Hannachi et al 2017). However, standard methods for identifying regimes are not designed to disentangle those dynamical drivers that are particularly informative of the local impact (Bloomfield et al 2021). Here, we introduce a modified variational autoencoder architecture (CMM-VAE) to target the identification of circulation regimes to a local impact while still obtaining a complete representation of the atmospheric phase space. In an application to extreme precipitation over Morocco, we show that the CMM-VAE method identifies regimes that are more informative of the target variable while maintaining their own predictability in S2S hindcasts and teleconnection to large-scale drivers.
Registration: xaidaproject@gmail.com
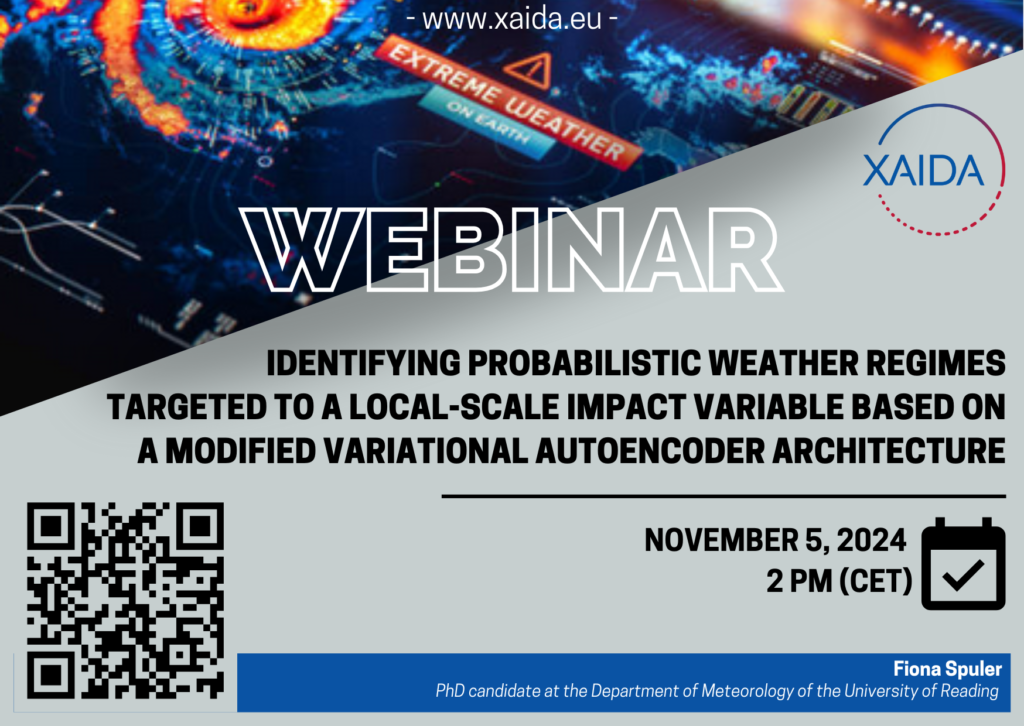