WP4 • Causal inference for detection and attribution of extreme event pathways
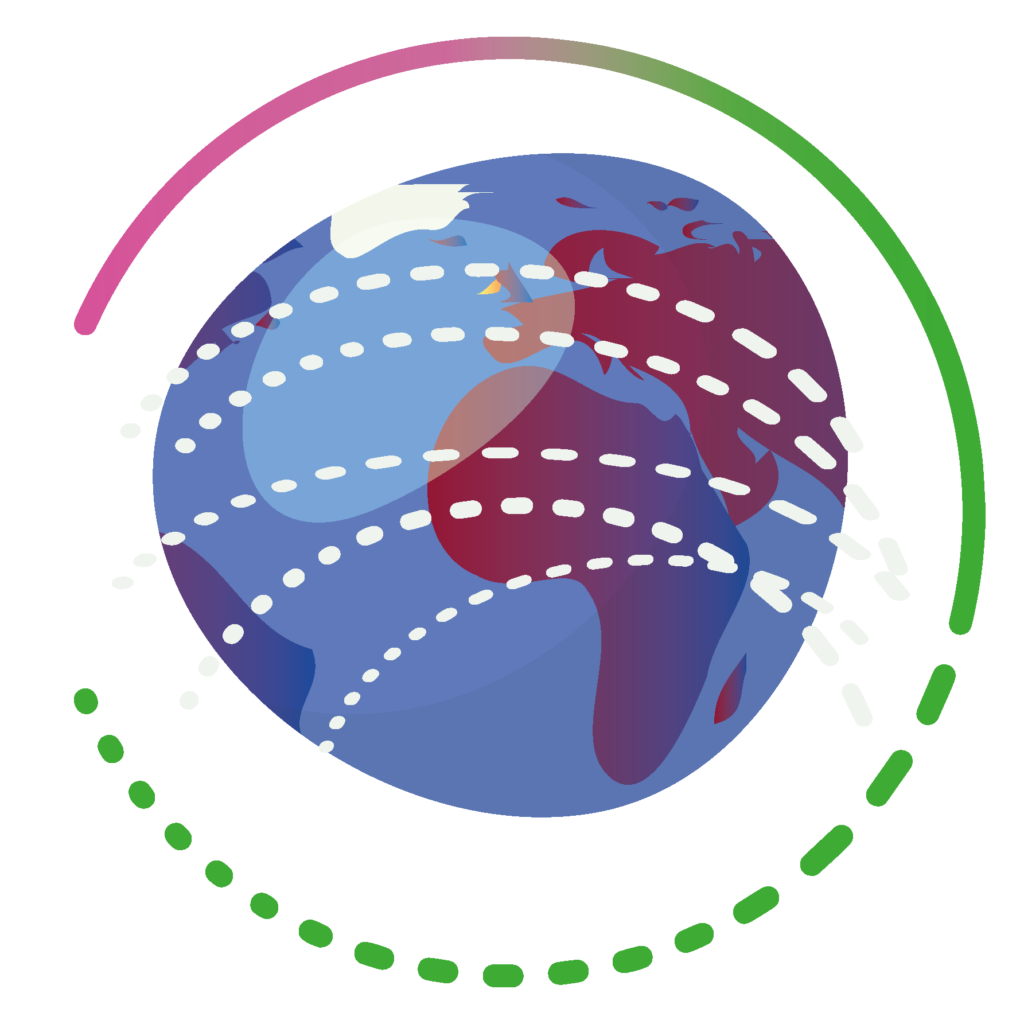
WP4 develops methods to identify the physical drivers and pathways leading to extremes using novel machine learning techniques within a causal inference framework. This way the role of climate change on each of these drivers can be assessed, adding credibility to attribution statements.
Moreover, the representation of drivers and pathways in climate models can be validated and conditional attribution statements made. Conditional attribution statements are storylines, and are an established form of evidence in the IPCC WGII (adaptation) context. The analysis of extremes and their drivers requires significant advancements of current causal inference methods.
This WP addresses three main challenges:
(i) heterogeneous drivers (e.g. mixed continuous and discrete or categorical variable types) as emerging from the way extreme events are reconstructed in WP3,
(ii) compound or synergistic drivers where only the combination of certain states in two or more drivers leads to an extreme in a target variable,
and (iii) non-stationary relations.
The improved causal inference methods also allows us to evaluate the relevance of individual pathways and how these pathways differ among scenarios. This functionality is added to existing causal inference tools in a generic way to be applicable to event types in WP6 and WP7.