WP3 • Advanced ML methods for detection, localization and characterization
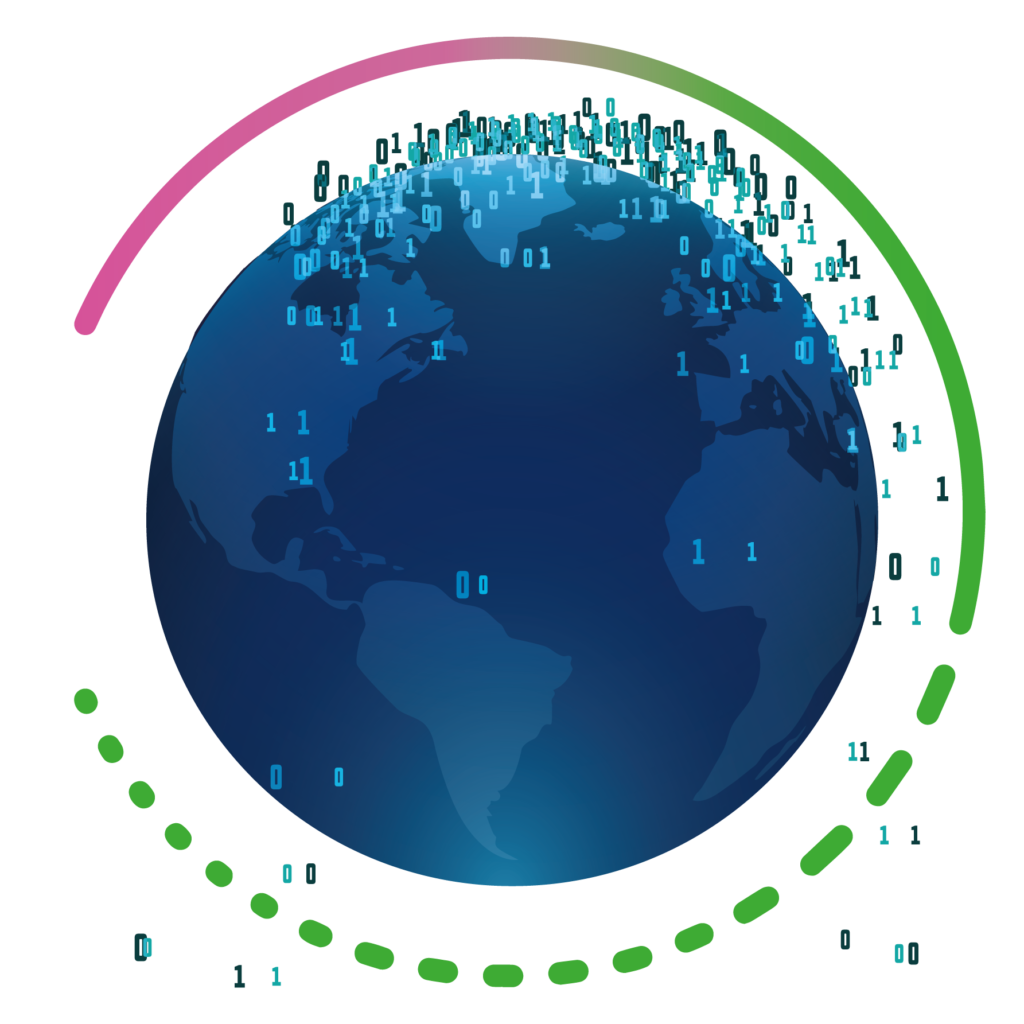
Lead: G. Camps-Valls • M. Reichstein
ECS* lead: Ana Bastos (MPG), Nora Lindscheid (MPG) & Miguel-Ángel Fernández-Torres (UVEG)
WP3 is developing the detection and characterization of extremes with advanced machine learning (ML) algorithms. It develops and applies new machine learning approaches around three main inference paradigms: deep learning, generative models and Bayesian (probabilistic) nonparametric models.
After some adaptation, the algorithms will be generic enough to be applicable to all event types considered in XAIDA, to (i) detect, localize and characterize extreme events in the climate system in a data-driven way: detect/delineate in space and time the event, as well as to quantify statistics about the event’s frequency, intensity, spatio-temporal distribution and persistence; (ii) associate impacts on biosphere and society with the characteristics of the extreme events. i.e. learn ML mappings of impact as a function of extreme event characteristics and other biospheric and societal conditions.
This will allow us to predict socio-economic impacts, e.g. infrastructure impacts, economic loss, or population of concern from the previous characteristics of the extreme; and (iii) infer trends in extreme events and their characteristics, as well as their impacts useful to distinguish impacts driven by trends in extreme events, physical and societal conditions.
*ECS = Early Career Scientists