SUMMER SCHOOL: ARTIFICIAL INTELLIGENCE FOR DETECTION AND ATTRIBUTION OF CLIMATE EXTREMES
Dates: 20 June – 2 July 2022
Location: ICTP, Trieste, Italy
Format: Hybride (face-to-face or virtual)
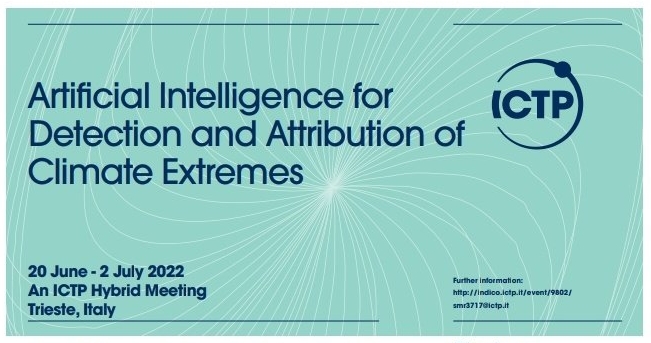
ORGANISATION
As presented at the Kick-off meeting in September 2021, Davide Faranda and Erika Coppola were the leaders of the Summer School organisation. The programme has been elaborated with the volunteer speakers and with the strong support of Aglaé Jézéquel. As per the logistic and administrative side, Manon Rousselle and the ICTP support team have been deeply involved in the budget changes discussion, legal details and practical organisation.
The main topics were Dynamics and Thermodynamics of Extreme Events in a Changing Climate, Statistical and Artificial Intelligence Methods for the attribution of extreme events but also Communicating Attribution Results to the general public, stakeholders and other scientists in an exact although non specialist language.
The scientific committee, since the beginning, was composed by:
Emanuele BEVACQUA (UFZ, Germany)
Erika COPPOLA (ICTP, Italy)
Dim COUMOU (VU Amsterdam, Netherlands)
Davide FARANDA (LSCE-IPSL, CNRS, France)
Aglae JEZEQUEL (LMD, ENS Paris, France)
Robert VAUTARD (LSCE-IPSL, CNRS, France)
Mathieu VRAC (LSCE-IPSL, CNRS, France)
Pascal YIOU (LSCE-IPSL, CEA, CNRS, France)
The objectives were clearly formulated in the proposal of the project. First of all, to share and devise techniques to tackle the problem of attributing meteorological extreme events to climate change. Secondly, to raise awareness of the possible consequences of changing climate on vulnerable societies and ecosystems, often located in developing countries, through rigorous attribution. Finally, the open source and global nature of the techniques taught in the school could then be used by scientists in developing countries independently for local attribution studies.
After several consultations with the governing board meeting of the project and the potential speakers and the ICTP teams, it was decided that the Summer School would take place from June 20th to July 2nd. The location and format were also largely discussed and the selection of the participants with their profile was closely followed.
- Dynamics and thermodynamics of extreme events (including heatwaves, cold spells, severe convective events, tropical and extra-tropical cyclones, compound extremes at different scales)
- Statistical tools for extreme event attribution (including rare events algorithms, compound climate extremes, storylines, casual inference, downscaling and bias correction)
- Machine Learning for extreme event attribution (including phyisics-aware machine learning, explainable artificial intelligence for climate sciences, casual discovery algorithms for extreme events)
- Outreach and communication training (including a creative writing workshop, communication of extreme event attribution to the general public, school outreach activities and outreach videogames)
The choice of ICTP was made before the beginning of the project thanks to the interest the Institution has in this training school, the facilities and the opportunity of the logistic and administrative support.
Trieste is very accessible by train and plane, the main building of ICTP well connected by train and bus with the station and the airport. The Summer School has been held in the Adriatico building near the Castle of Miramare at Grignano, with a great sea view. Comfortable conference rooms and labs were booked for the School.
ICTP offered full technical support for the hybrid format and 10 000 EUR, especially to help fund early career scientists (ECS) and researchers from developing countries to join the Summer School. The XAIDA budget covered the other 2⁄3 of the total costs. The costs were dedicated to the students/attendees. The speakers and organisers had to fund their own travels and expenses. It was a hybrid format and as such, it has been decided that the afternoons and consequently all the training sessions, would be reserved for the in-person attendees only. This decision was due to the number of participants and for practical/technical reasons.
The ICTP capacity for an in-person event is 50-55 attendees and 10 to 15 speakers. In the end, the applications were so successful that there were 168 persons attending the Summer School in total: 11 speakers, 1 tutor, 8 directors2 and 148 participants, with 40% of women. 70 persons were on site (42%) and 98 online (58%).
For all the persons attending the Summer School, 51% came from developing countries, 8% from least developed countries and 41% from developed countries. In terms of geography, 21% of the participants came from Africa, 24% from Asia, 39% from Europe, 11% from Latin America, 4% from North America; the last percent was from Oceania and the Middle East (See Table 3 below).
The selection has been made over 255 applicants and 148 were selected to attend: 53 in person (36%), 95 online (64%). Most of the participants were PhD students, few had a Master Degree level only and a little fewer were Postdoc. Globally speaking they were all Early Career Scientists (ECS), mostly in the XAIDA networks.
Over the 46 answers of the evaluation form which represent 31% of the participants, we can have an overview of the profile of the attendees: 56,5% were PhD candidates, 26% Graduated students and 17,5% Post-doc. 30% of them were specialized in Modelling, 26% in Deep Learning, 24% in Attribution and the 20% other have multiple background such as Extreme events and climate litigation (previously MSc on attribution); Application of rare event algorithms on climate models; Civil engineering and Data science; Atmospheric and Climate Science; Geosciences; Tropical Meteorology; Climate variability and climate extreme; Atmospheric scientist.
74% of them attended the Summer School to learn more about AI and Deep Learning. 32% wanted to create a network in their field of specialty and 43% were interested in the lectures. 20% wanted to know more about the project. 2 people answered that they wanted to know more about attribution and extreme events but also modelling.
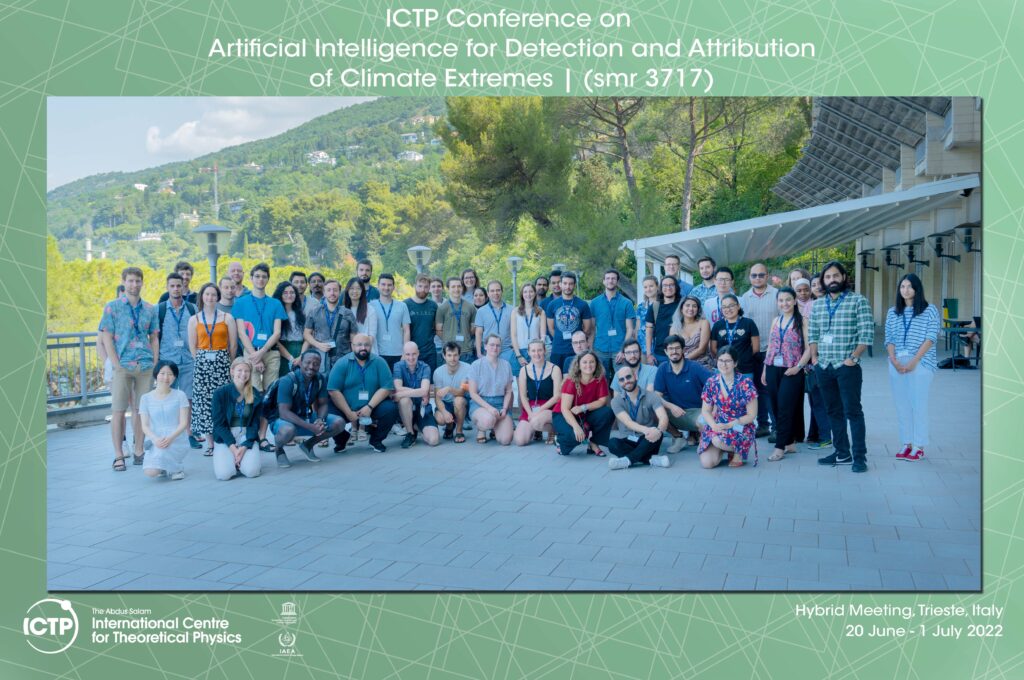
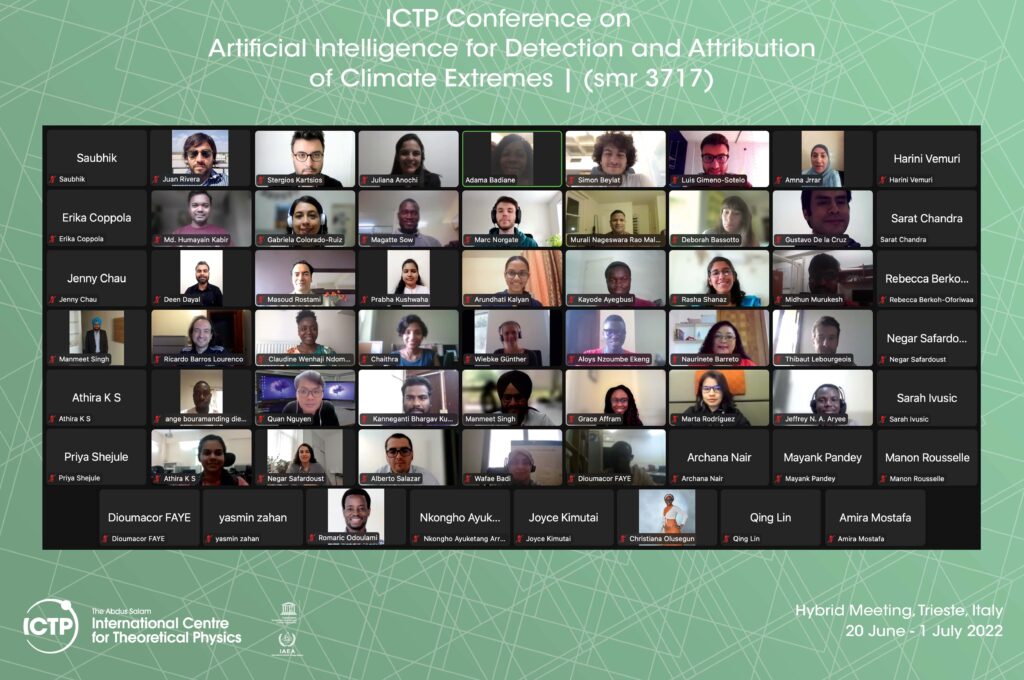
PROGRAMME AND MINUTES
After several last-minute changes due to personal issues for different speakers but also to transportation strokes at this time of the year in some parts of Europe, the programme was communicated very late to the attendees. The programme on the ICTP website was not completely updated so we chose to give a table to all the speakers and attendees. We will relate the minutes according to the days while separating the lectures from the training sessions.
MINUTES OF THE LECTURES
DAVIDE FARANDA – « PHYSICS LEARNING FOR THE ATTRIBUTION OF ATMOSPHERIC EXTREME EVENTS »
The first day of the summer school I presented an analogues-based methodology to perform extreme events attribution to climate change: this methodology is largely based on the use of dynamical systems theory which prescribes a precise mathematical way to look at the recurrences of a state in phase space (the space of all the variables of the system). This formalism is particularly useful in climate sciences because it allows to go beyond the detection of events similar to the one to attribute to climate change based on thresholds exceedances, it allows to perform attribution conditioned to a circulation leading to a specific extreme event. During the presentation I’ve also presented an application to the attribution of the Medicane Apollo, which is one of the XAIDA case’s study. At the end of the presentation, there was time to interact with the students that were very active in asking questions and suggesting also new line of research.
MIGUEL-ÁNGEL FERNÁNDEZ-TORRES – « SPATIO-TEMPORAL DEEP NEURAL NETWORKS FOR EXTREME EVENT DETECTION »
A review of the different deep learning methodologies for spatial, temporal and spatio-temporal modelling was done during the presentation, including Deep Neural Networks (DNNs), Convolutional Neural Networks (CNNs) and Recurrent Neural Networks (RNNs). These methodologies were introduced with the goal of extreme event detection, illustrated by following a case study about drought monitoring during the severe Russian heat wave in 2010. Moreover, the talk explained the different concepts applied during Hackathons 2 and 3 (e.g. convolutional and pooling layers, activation functions, optimization, backpropagation, etc.).
ERICH FISCHER – « QUANTIFYING AND UNDERSTANDING VERY RARE EXTREMES”
The keynote lecture on « Understanding and quantifying changes in very rare extremes » by Erich Fischer demonstrated that recent weather and climate extremes broke long-standing observed records by large margins. Many of these unprecedented extremes often led to very large socio-economic and ecological impacts due to a tendency to adapt at most to the highest intensities experienced during a lifetime or documented historically. This lecture discussed challenges and opportunities of understanding and quantifying record-breaking events and different methods to assess the potential for future events unseen in the observational record. The lecture reviewed different methodological approaches to produce physical storylines and different lines of evidence from model-based storylines and physical process understanding.
EMANUELE BEVACQUA – « COMPOUND WEATHER AND CLIMATE EXTREME EVENTS »
Compound weather and climate events are combinations of climate drivers and/or hazards that contribute to societal or environmental risk. Studying compound events often requires a multidisciplinary approach combining domain knowledge of the underlying processes with, for example, statistical methods and climate model outputs. Recently, to aid the development of research on compound events, four compound event types were introduced, namely (a) preconditioned, (b) multivariate, (c) temporally compounding, and (d) spatially compounding events. In this talk, I introduced compound events and guidelines on how to study different types of events. The talk was based on a paper where we consider four case studies, each associated with a specific event type and a research question, to illustrate how the key elements of compound events (e.g., analytical tools and relevant physical effects) can be identified. Furthermore, examples of studies on projections of other types of compound events were given. Overall, the insights of the presentation can serve as a basis for compound event analysis across disciplines and sectors.
MATHIEU VRAC – « STATISTICAL DOWNSCALING AND BIAS CORRECTION »
The keynote lecture on « Statistical downscaling and Bias correction of climate simulations » by Mathieu Vrac first presented the needs for post-processing climate simulations before investigating climate change impacts. This lecture reviewed the various categories of statistical methods for downscaling (SD) and/or bias correction (BC), including discussions about multivariate aspects and methods.
Challenges and opportunities for developments and applications regarding extreme events were also discussed. The question of the stochasticity in SD and BC results, as well as potential developments regarding “physically-based” SD or BC methods (e.g. via large-scale circulation inclusion and modelling) were also parts of the presentation and discussed with the audience.
SEBASTIAN SIPPEL – « DYNAMICAL ADJUSTMENT AND DISTRIBUTIONAL ROBUSTNESS FOR D&A »
Abstract: Internal climate variability fundamentally limits short- and medium-term climate predictability, and the separation of forced changes from internal variability is a key goal in climate change detection and attribution (D&A). In this talk, I discuss the suitability of incorporating statistical learning techniques to identify forced and internal climate signals from spatial patterns of climate variables.
First, I demonstrate that statistical learning techniques can be used to characterize and remove the influence of internal atmospheric variability on target variables such as temperature or precipitation (“dynamical adjustment”), which enables a better characterization of the forced thermodynamic response of climate change. It is shown that well-known climate features such as the late 1980’s abrupt climate change in Switzerland can be explained by such techniques as a combination of internal variability and externally driven changes.
Second, I introduce a detection approach using climate model simulations and a statistical learning algorithm to encapsulate the relationship between spatial patterns of daily temperature and humidity, and key climate change metrics such as annual global mean temperature. Observations are then projected onto this relationship to detect climatic changes, and it is shown that externally forced climate change can be assessed and detected in the observed global climate record at time steps such as months or days. I discuss how these approaches can be extended to address key remaining uncertainties related to the role of decadal-scale internal variability (DIV). DIV is difficult to quantify accurately from observations, and D&A requires that models simulate internal climate variability sufficiently accurately. I show that recently developed statistical learning techniques, inspired by causality, allow to identify the externally forced global temperature response, while increasing the robustness towards different representations of DIV (via an explicit robustness constraint). The fraction of warming due to external factors, based on these optimized patterns, is more robust across different climate models even if DIV would be larger than current best estimates.
JAKOB RUNGE – « CAUSAL INFERENCE FOR CLIMATE SCIENCE »
The presentation of WP4 at the Summer School, held by Jakob Runge, was a one-hour presentation about the general framework of causal inference with a focus on applications to climate science and especially extreme events. The causality framework can answer causal questions in a setting where randomized experiments are not possible. This is particularly the case in climate science, where most data are observational or from simulations. In the talk, Jakob presented why and how causal inference methods have the potential to deepen the understanding of extreme events. The causality toolbox, which was introduced in the talk, can help find drivers of extreme events by going beyond mere association. However, using such methods on climate data brings a set of challenges. Among these are the high dimensionality of the data, non-linear dependencies, or unobserved variables. These and more were also presented in the talk. A short discussion followed after the talk, where attendees posed questions about specific use-cases where causality could be used.
AGLAÉ JÉZÉQUEL – « EXTREME EVENT ATTRIBUTION”
This presentation was two-part. The first part explained the different techniques used to perform extreme event attribution. The class covered topics such as : how to define the event of interest, the concepts of counterfactual and factual worlds, storyline and risk-based approach, the attribution of impacts (beyond weather hazards), and compound events attribution. The second part focused on the uses and usefulness of extreme event attribution. It was the occasion to discuss with the students about the challenges of interacting with stakeholders, and of how hard it is to evaluate the added value of scientific information in decision making. The discussion with the room was lively.
GUSTAU CAMPS-VALLS – « PHYSICS-AWARE MACHINE LEARNING »
Most problems in Earth sciences aim to do inferences about the system, where accurate predictions are just a tiny part of the whole problem. Inferences mean understanding variables relations, deriving models that are physically plausible, that are simple parsimonious, and mathematically tractable.
Machine learning models alone are excellent approximators, but very often do not respect the most elementary laws of physics, like mass or energy conservation, so consistency and confidence are compromised. I will review the main challenges ahead in the field, and introduce several ways to live in the Physics and machine learning interplay. Physics-aware machine learning models are just a step towards understanding the data-generating process, for which causality promises great advances. I’ll review some recent methodologies to cope with it too. This is a collective long-term AI agenda towards developing and applying algorithms capable of discovering knowledge in the Earth system.
https://arxiv.org/pdf/2010.09031.pdf
https://arxiv.org/abs/2104.05107
DIM COUMOU – « SPATIALLY COMPOUNDING SUMMER EXTREMES »
On the morning of Friday 24th of June, Dim Coumou gave a 1-hour lecture on spatially compounding summer extremes, including simultaneous heat waves in different regions in the northern hemisphere mid-latitudes. Such simultaneous extremes, driven by large-scale waves in the jet, provide substantial risks for global food production as the affected regions are important breadbaskets (US mid-west, western Europe, Russia/Ukraine and east Asia). Coumou introduced the different hypothesized drivers of quasi-stationary waves in the summer jet. Next, he presented a series of studies using both climate models and data-driven methods (in particular causal discovery analyses) to quantify the relevant importance of these hypothesized drivers, and how anthropogenic global warming (AGW) can affect those. He showed that AGW to date has likely led to a weakening of the summer jet and storm tracks, something that is also well-captured by the latest generation of climate models (CMIP6). However, there is still substantial uncertainty into how AGW affects quasi-stationary waves. Coumou highlighted the usefulness, and limitations, of causal discovery methods in teleconnection and extreme weather research.
SIMON KLEIN – « CLIMATE CHANGE EDUCATION – CO-WORKING ON SCHOOL ACTIVITIES AND PROJECTS »
As required by the climatologist community and IPCC experts as well as stated in the Paris Agreements, education is a key element to tackle climate change and take collective climate action. The Office for Climate Education (OCE), a UNESCO category 2 center, affiliated with Sorbonne University and IPSL is a major actor in contributing to climate change education via producing high quality pedagogical resources, and facilitating teachers training around the world.
OCE is one of the XAIDA WP2 members, and along with the MET Office, is contributing to the dissemination of XAIDA findings and research by creating sets of lesson plans, short videos and teachers training sessions on extreme events, involving the XAIDA community. In Trieste, Simon Klein presented the engagements of the OCE contributions to the XAIDA project, and the importance of working with young scientists in design pedagogical tools, but also to acknowledge, in the process, the importance of emotions that such results can trigger to the learners, such as the eco-anxiety.
DAVIDE FARANDA – « CHALLENGES IN DETECTION & ATTRIBUTION OF TROPICAL AND MEDITERRANEAN CYCLONES »
On the first day of the second week I present the challenges in attribution of tropical and mediterranean cyclones to climate change: indeed in recent years, multiple groups started to pursue attribution studies for specific convective events mostly focusing on tropical cyclones or tropical cyclone seasons. The goal of attribution is to evaluate the role of climate change in modifying the intensity and frequency of specific extreme weather events. The students were particularly interested in the limitations previously in observing and simulating tropical cyclones which prevent from directly attributing a severe cyclone to climate change: this would ideally require two large sets of high-resolution cyclones simulations with and without climate change. We had several follow-up discussions on this topic.
DAVIDE FARANDA – OUTREACH GAME “CLIMARISQ”
On the first day of the second week we also played ClimarisQ. ClimarisQ is a smartphone/web game from a scientific mediation project that highlights the complexity of the climate system and the urgency of collective action to limit climate change. It is an app-game where players must make decisions to limit the frequency and impacts of extreme climate events and their impacts on human societies using real climate models. The students gladly play the game and made several observations to improve its dynamics: most of their comments are already implemented in a new version of the game that should be out at the beginning of next year.
TED SHEPHERD – « BRIDGING PHYSICAL HYPOTHESES AND THEIR STATISTICAL ANALYSIS THROUGH CAUSAL NETWORKS »
Ted Shepherd gave a lecture prepared by Marlene Kretschmer entitled ‘Bridging physical hypotheses and their statistical analysis through causal networks’. The lecture introduced basic aspects of causal inference, meaning the quantification of known causal relationships, illustrated through application to atmospheric teleconnections. Causal inference is to be distinguished from learning causal relationships from data, known as causal discovery. Causal inference can be applied where there is already a large amount of expert knowledge available concerning physical hypotheses. This is the case with atmospheric teleconnections, which have a strong influence on the statistics of extreme weather and climate events. The scientific challenge is that of quantifying the causal linkages in the presence of multiple interacting factors, non-linearity, and non-stationarity. This approach also allows for a meaningful common language between dynamicists and statisticians, which is important for combining different lines of evidence. The lectures illustrated basic concepts such as blocked and open pathways of influence, common drivers, mediators, and common effects, and how these determine how to control for confounding influences in order to properly quantity causal effects. The students found the lectures very clear and pedagogical.
ROBERT VAUTARD – « STATISTICAL PRACTICE IN EXTREME EVENT ATTRIBUTION »
Robert Vautard gave a lecture explaining the principles of probabilistic event attribution, which rely on defining a class of events, such as the occurrence of a threshold exceedance, and analyzing whether such an event would have more or less probability to occur in a counterfactual climate altered by human activities. He explained also how such probabilities can be calculated from statistical modeling, and then followed by describing how such an approach is handled in operational attribution studies such as made in the “world weather attribution” network. He went on describing the protocol underpinning these activities, which allows a quasi-operational approach. He then provided several examples of event attribution analyses and their interpretations: extreme rainfall over the Seine river basin, drought in Madagascar, wind storm in Western Europe, growing-period frosts in France, Pacific North American heat wave. He then developed one of the main pending issues: why do models appear to underestimate heat waves in Western Europe? The question of model evaluation is absolutely crucial for attribution.
He concluded by describing main issues and bottlenecks when carrying out rapid attributions.
TED SHEPHERD – « STORYLINES »
Ted Shepherd gave a lecture entitled ‘The storyline approach to the construction of useable climate information at the local scale’. Storylines are self-consistent articulations of past or plausible future events or pathways, based on causal reasoning. They can be regarded as instantiations of the range of possibilities represented in causal networks, and thus are closely related to causal inference.
Storylines are particularly useful for representing combinations of epistemic (or systematic) and aleatoric (or probabilistic) aspects of uncertainty, which are not describable using standard (i.e. frequentist-based) statistical methods still widely used in climate science, such as null hypothesis significance testing. The lecture covered a range of aspects including the motivation for storyline approaches to regional climate risk, different kinds of storylines, the links to causality, the pitfalls of aggregation, the benefits from conditionality, and the interpretation of storylines within Bayesian statistics. In the days following the lecture, Ted had one-on-one discussions with many of the summer school participants. The connection with probability was perhaps the most common topic of discussion. Probabilities are appealing, but coming to terms with what are fundamentally subjective probabilities – as epistemic uncertainties necessarily are – is difficult for climate scientists.
ERIKA COPPOLA – « HEAVY PRECIPITATION EVENTS AND CONVECTION PERMITTING MODELLING »
The definition and concept of extreme precipitation has been introduced and several example presented to illustrate how the definition of extreme precipitation may be different in space and time and also how different can be the consequences in term of impacts. A brief recap of what is convection has been presented and which are the main limitations in modelling convection in the state of the art climate models where this process is parametrised and how this is relevant for the uncertainty of climate model projection. Therefore why the increase in resolution is needed to be able to explicitly model the convection and the state of the art of convection permitting regional climate modelling technique has been presented. Which are the tools and methodologies for this kind of dynamical downscaling, like for example which equations are needed for the model core since the hydrostatic approximation cannot be used anymore, which are the microphysical scheme that can be used for an explicit representation of convection (5-class microphysics for example), which are the possible nesting techniques to be used.
The need of very high computational resources and storage space has been explained for such kind of simulations and the discussion on the limitation of the model physics introduced. A summary of the main finding was presented showing what is possible to improve by simulating the climate at such resolution spanning from the better representation of spatial patterns and variability of precipitation at daily and hourly time scales, to the improved the representation of hourly frequency and intensity and summer diurnal cycle, representation of extreme events and reduction of model uncertainty.
The main community effort projects like EUCP and CORDEX-FPS Convection have been introduced and the main findings of the first ensemble of convection permitting climate simulations illustrated for both the present day validation and the climate projection. A summary of the key finding of similar extra European CP projects was also reported and discussed.
PASCAL YIOU – « SIMULATION OF RARE CLIMATE EVENTS »
Pascal Yiou made a lecture on the statistical methods to simulate extreme events. The goal was to provide the necessary information so that the students to facilitate a « hands on » experience in the afternoon.
The general mathematical background was defined and illustrated with examples. Care was taken to expose the mathematical formulation of the scientific questions and the strategies to treat them in a pedestrian fashion. This lead to general definitions of « rare event algorithms ».
A brief introduction to stochastic weather generators (SWGs) was proposed. SWGs are convenient tools to simulate very large ensembles of trajectories. The lecture was illustrated by a case study around the heatwave that struck north western US and Canada in the summer 2021. This illustrated how climate change affects the features of the most extreme events.
GABRIELE MESSORI – « LINKING LARGE-SCALE DYNAMICS TO REGIONAL EXTREMES »
Extreme climate events have multifarious detrimental impacts on both society at large and natural systems. The impacts of the extreme events themselves are often on a local to regional scale, but the physical processes that originate them can occur on much larger scales, up to several tens of thousands of kilometers. In this presentation, I review some of the large-scale atmospheric drivers of regional extremes, focusing on elements such as blocking, Rossby waves and jet streams. I also touch upon the role of these large-scale drivers in synchronizing extreme events across geographically remote regions.
THE TRAINING SESSIONS
HACKATHON 1 (20 JUNE) – « STATISTICAL METHODS FOR EXTREME EVENT ANALYSIS: HANDS ON » (DAVIDE FARANDA & AGLAÉ JÉZÉQUEL)
In the afternoon Aglaé Jézéquel and Davide Faranda performed an attribution exercise: the exercise consisted first on explanations on the statistical basis to perform extreme event attribution based on extreme value theory, then on a hands-on phase where the students could perform the attribution of extreme events using pre-prepared data. One of the most important outcomes of this exercise session was the fact that three of the students, namely Cadiou, Noyelle and Malhomme brought to publication their exercise (https://doi.org/10.1007/s13143-022-00305-1)
HACKATHON 2 (21 JUNE)- « DEEP LEARNING FOR SPATIO-TEMPORAL DROUGHT FORECASTING » (M.-Á. FERNÁNDEZ-TORRES)
In this hackathon, the students got a brief introduction to the use of PyTorch [Paszke et al., 2019] for the development of deep learning-based architectures [Goodfellow et al., 2016]. To that end, they had to implement and evaluate a multilayer perceptron for drought forecasting as a multi-class classification task in the United States [Minixhofer, C. et al., 2021].
The hackathon was conducted in a tutorial fashion using a Jupyter notebook, leaving time for the students to try to solve the different activities proposed. First, the database, its loading, normalization, and visualisation were presented. Then, the neural network was implemented, together with the loop for its execution during the training stage. Moreover, early stopping, which is a core strategy to select the best model in this phase, was reviewed. Last but not least, the confusion matrix was explained as an evaluation tool during the test stage of the system. Given the long duration of the hackathon, activities regarding early stopping and the confusion matrix were finished during Hackathon 3 session, which was directly related to it.
Students who chose the hackathon as a final project worked on the improvement of the training stage of the developed model and its extension on the basis of long short-term memory units, being able to outperform the initial baseline model.
HACKATHON 3 (22 JUNE) – « PRECIPITATION DOWNSCALING USING DEEP NEURAL NETWORKS » (M.-Á. FERNÁNDEZ-TORRES)
In this hackathon, the students developed a convolutional neural network (CNN) architecture [Goodfellow et al., 2016] for precipitation downscaling [Wang, J. et al., 2021] using PyTorch [Paszke et al., 2019]. The hackathon was conducted in a tutorial fashion using a Jupyter notebook, leaving time for the students to try to solve the different activities proposed. Given that the structure of the hackathon was similar to the one followed in Hackathon 2, its remaining activities (early stopping, confusion matrix) were solved first. Then, a baseline CNN architecture for precipitation downscaling was implemented, following the same procedure as in Hackathon 2 for training and evaluation purposes.
Students who chose the hackathon as a final project worked on the improvement of the developed model by changing its complexity (number of layers and filters per layer) and attempting to incorporate attention mechanisms.
CREATIVE WRITING WORKSHOP (23 JUNE) – (AGLAÉ JÉZÉQUEL)
Participants were asked to work in pairs. They started by a « silence bal » where they were supposed to only communicate with their partner in written form. They were then asked to think about what had fictional potential in their research, and to come up with pitches of short stories they could write about it. Some of them wrote short stories after the workshop. A network of potential writers was also established but I have lacked time to animate since the end of the school. There’s still potential for this network to evolve, and maybe to collect stories from the participants to the training school in the coming months.
OPEN DISCUSSION ABOUT HUMANITARIAN WORK, INTERESTS, NEEDS (27 JUNE) – (RCCC TEAM)
At the Summer School, we virtually facilitated an interactive session that covered topics that included the communication of attribution findings, the vulnerability and exposure context, and the potential impact of attribution studies on decision-making. We reflected that despite not being able to attend in person, we found that students were extremely interested in learning about our experience as a humanitarian organization working with attribution scientists and potentially using the findings. We engaged the students in a game and used the game mechanism to simulate consequences and rewards for making decisions based on attribution findings. The students asked thoughtful questions about the utility of different findings and discussed how they could be complemented or contextualized for different end-users. Overall, we found it a valuable experience to engage with a new generation of attribution scientists on critical questions that they will need to engage with as they do more of this work.
HACKATHON 4 (28 JUNE) – « ADVANCED METHODS FOR ATTRIBUTION, INCLUDING VULNERABILITY AND ADAPTATION ASSESSMENT » (ROBERT VAUTARD)
The activity of Hackathon 4 was to carry out in practice a WWA-type of attribution, applied to the case of the ongoing June 2022 heat wave. The exercise, made collectively by the group of students, led to a published XAIDA brief on the heat wave and is available on the XAIDA website: https://xaida.eu/early-2022-heat-waves-in-europe/
The exercise was organized in about 10 groups, each collecting data and exploring numbers by using the Climate Explorer tool https://climexp.knmi.nl developed by the KNMI partner of XAIDA. Each group was assigned to a subregion of Europe and studied how exceptional the event was and what was the change in statistical properties due to climate change.
HACKATHON 5 (29 JUNE) – « CONVECTION PERMITTING CLIMATE MODELLING DATA ANALYSIS » (EMANUELA PICCHELLI)
The activity of Hackathon 8 dedicated to atmospheric convection had the purpose to give the students an overview on how this phenomenon can be represented through regional climate models (RCM).
Conceptual aspects about atmospheric convection and its representation within the RCMs have been sketched, giving an overview on the advantages of simulating at the km scale for the reduction of model uncertainty and the proper representation of heavy precipitation events (HPE).
Then an exercise session followed. Observational and model data were available to the student and couple of exercise were proposed:
1. Analysis of extreme precipitation events case studies based on observations and assessment of ERA-Interim reanalysis products in reproducing such kind of events, whose purpose was to make the student aware about the concept of HPE and how this can or can not be represented in an area limited model.
2. Extended analysis over a statistical sample of extreme precipitation events by means of an appropriate statistical procedure. The exercise was shaped to make the students to:
a. Build a statistical analysis of precipitation. Example scripts were available, but also self-built analysis was welcome. A 10-years ICTP model dataset of precipitation was available for the scope.
b. Select the most appropriate statistical indices among several for the analysis of HPEs within observations and RCMs at different resolutions and eventually draw added value of models at the km scale. Ready statistical data were available for framing a multi-model analysis in comparison with multi-
observational dataset.
c. Select the most appropriate methodology to analyse the model spread/uncertainty for the above selected quantities. Two to three student groups were mainly interested in this activity and successfully performed the proposed analysis. Some of them were able to give a final presentation of their results.
HACKATHON 6 (30 JUNE) – « SIMULATING THE MOST EXTREME EVENTS » (PASCAL YIOU)
Pascal Yiou prepared computer codes and data sets on github, so that the students could reproduce the results presented in the lecture (made in the morning) and experiment by themselves on other regions (e.g. France, Europe), to simulate either hot or cold events. The students were able to download the prepared material from github and follow the training on the data sets. Two students (Camille Cadiou and Robin Noyelle) helped their fellows in bridging the R format into python (as most attendants were more familiar with python than R). In the end, one group chose to work on the hackathon of simulating extreme heatwave in the Iberian Peninsula. The results were promising, and were harbingers of the heatwave to come in that region during the summer.
This lecture/hackathon was the last of the school. The attendants hence had little time to work on that topic. I was immensely satisfied that a group of students was able to master the concepts and tools that were exposed to produce an interesting preliminary result in just a few hours.
FEEDBACK AND EVALUATION
The evaluations and feedback collected concern the participants and the lecturers. Two questionnaires were spread to identify better the expectations and improvements needed from both sides. We analysed first the evaluation and then made a synthesis of the feedback.
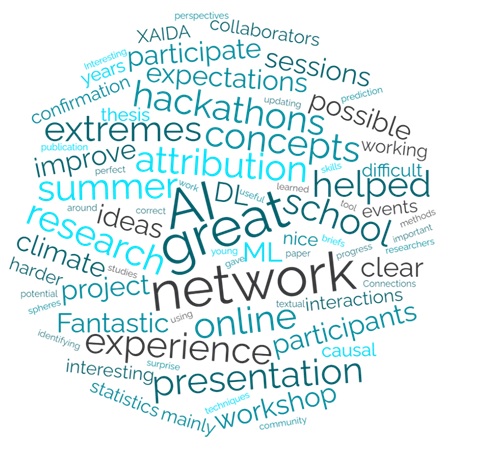
EVALUATION OF THE SUMMER SCHOOL
Generally speaking, organisers, speakers and attendees were satisfied during the Summer School and everything went well at the logistic level, despite last minute transportation issues and a small Covid19 cluster during the second week of the School.
THE SPEAKER’S PERSPECTIVE
A little more than 50% of the speakers answered the evaluation form, and 89% of them were making lectures/training exercises in-person.
Generally speaking it was a good organisation with 89% of the answers ranking the organisation between 7 and 104, one answer remains neutral (rank 5). 50% of the answers show that they are extremely satisfied by the choice of the location, and the other answers are rather satisfied (rank between 7 and 9 over 10).
Regarding the programme and duration, speakers are generally also satisfied, regarding the balance between AI and Attribution. The ranking is however more mixed with 33% between 6 and 7 (satisfied, not extremely satisfied) for the balance, as well for the duration. We can see in the feedback part that duration and balance between AI and Attribution were at the center of the discussion after the Summer School.
THE ATTENDEES PERSPECTIVE
We spread an evaluation form a few weeks after the Summer School to the attendees. We got 46 answers which means the evaluation represents about 31% of the attendees. 93,5% of them have seen their expectations satisfied by the Summer School and 100% would attend another XAIDA training school. We can conclude that, generally speaking, the attendees were very satisfied with this Summer School.
Generally speaking, 74% of the attendees were very/extremely satisfied by the programme (rank between 8 and 10) and 26% were rather satisfied (rank between 5 and 7). Some of the detailed answers express that attribution and AI were presented separately and there was no clear link between those topics. The organizers will pick up this point for the planned second XAIDA summer school.
The duration was extremely fine according to the ranking (11% rather agreed vs. 89% very satisfied). The balance between AI and attribution is experiencing more mixed feelings. Even if 71,7% are very satisfied, 2% are not satisfied, 4% are neutral and 21,8% are rather satisfied. The individual feedback from the attendees reveal that two weeks may be too long or too intense according to the programme.
We experienced a lot of issues with the budget transfer and the organisation (invitation, official answers, etc.) came very late to the attendees. It was very uncomfortable and we apologized for that. That can explain why 17,5% are neutral or unsatisfied regarding the organisation and the internal communication of the Summer School.
We have asked the attendees about the gender balance of the attendees during the Summer School. 32,6% are extremely satisfied, 45,7% are satisfied, 17,4% are rather satisfied, 2,2% remain neutral while 2,2% are clearly not satisfied. Selection has been made as inclusive as possible regarding gender, ethnicities and background. The evaluation of the gender balance of the speakers is more mixed with 24% very satisfied, 30,4% satisfied, 28,2% rather satisfied, 18% neutral or unsatisfied.
FEEDBACK
This synthesis has been made from the comments made to the questionnaires but also several discussions after the Summer School during internal XAIDA meetings were speakers and participants were free to express their feelings.
SPEAKERS POINT OF VIEW
At the occasion of a general meeting for all the XAIDA members available, we had a free and healthy discussion about the Summer School with a lot of feedback. Generally speaking, the lecturers and organisers were positively surprised by the level of the students and the environment created at the Summer School. Participants were really involved and felt free to ask all the questions they had. There were no imposed visions or methodologies, the networks and groups formed very quickly, mostly thanks to ice breaker activities organised by Aglaé in the first days. Hackathons were very much appreciated by the participants. For next summer school, maybe having more connection with the level of the participants, there was also a lack of balance between attribution hackathons and AI based ones. Lots of students asked for an introduction to the AI techniques. Hybrid format was very smooth and the ICTP team was very good.
Moreover, they made high quality final presentations. The last day they presented works on attribution or work with the convection permitting data or they also worked with the deep learning methodology.
They made nice projects that could be used for WWA or papers. We have a strong base of new
generation of attribution scientists or machine learning methodology to work with during the XAIDA project and even after.
ATTENDEES POINT OF VIEW
A recurrent point of view is that networking and meetings are more difficult since the pandemic; for example with the XAIDA kick-off meeting online. It was a very needed opportunity to have a chance to meet and discuss with people. The Summer School was quite intense with the lectures in the morning and the hackathon in the afternoons especially since they had to work on their projects the rest of the time.
Most of the negative feedback appears to come from online attendees for not being able to take part in the training session. It was indeed too complicated to include them for all the training sessions, due to the huge number of people but also time difference for some of them or simplier due to equipment.
Some attendees suggested that better communication, from the very beginning, could have avoided the negative feeling. Some people explained that it was sometimes too hard to follow on some topics, such as ML training sessions for AI beginners. The XAIDA members agreed after the Summer School that a better connection between lectures and hackathon and the level of the students/attendees would have made things clearer. Most remarks came around the need for more balance between AI hackathons and attribution ones – more generally speaking they asked for more AI hackathons but the attendees also requested more free time to build their projects. However, a misunderstanding has been made: the attendees were not supposed to attend all the training sessions but only those they wanted.
CONCLUSION
In terms of objectives, speakers and organisers agree that we have reached the main purposes of the School: to share and devise techniques to tackle the problem of attributing meteorological extreme events to climate change and, also, to raise awareness of the possible consequences of changing climate on vulnerable societies and ecosystems, often located in developing countries, through rigorous attribution. Papers, briefs and networks are growing and developing far beyond the Summer School itself.
Several types of networks were built during the Summer School. Thanks to no imposed visions or methodologies and the in-person event; for some of the students it was actually new because of the last years that have seen all classes and seminars and conferences remote and most of the time the distance cut the debate. There have been a lot of interactions and nice discussions among speakers too. The networks and groups formed very quickly, even friends, and people easily started to work together despite their institutions or countries.
On one hand we had the XAIDA Early Career Scientists (ECS) network and on the other hand, the external (but closed) networks. The XAIDA ECS network was successfully created during the Summer School and continues to grow with the new recruitments in the project, especially through a Slack channel (everybody is welcome to join), networking events, seminars on topics that concern the Young scientists work. More than 13% of the attendees explain that the main gain from the Summer School was the network they were able to make during this time. Most of the feedback regarding the building networks came from people outside the XAIDA project.
The social media network has experienced a huge growth thanks to the Summer School and XAIDA is now known from all over the world, at the scientific level.
We can say that another objective has been reached: the open source and global nature of the techniques taught in the school could then be used by scientists in developing countries independently for local attribution studies.
So far, papers and networks are growing up. One paper is already published: Cadiou, C., Noyelle, R., Malhomme, N. et al. “Challenges in Attributing the 2022 Australian Rain Bomb to Climate Change”. Asia-Pac J Atmos Sci (2022). https://doi.org/10.1007/s13143-022-00305-1
One successful brief (science fact-sheet) has been posted on the XAIDA website after the Summer School[1]. It is a study of the early heatwave and the attribution to climate change. Initiated by Robert Vautard in his hackathon on Tuesday 28 June, the writing has been done by Tamara Happe with the help of Robert Vautard and the other attendees of the hackathon.
Seeing the enthusiasm around this Summer School, it has been decided that we will organise another event such as this one. Most speakers and organisers suggest a 1-week duration. 2023 will probably be too short, 2024 could be better, especially regarding the fundings. Sonia explains that it could also work with the preliminary results in 2024.
We could think about a workshop/school online or even a small in-person group next year. Maybe focus on machine learning or else. Ted proposes to organise in each country/university/partner small groups in person and to be all hybrid for the lectures for example.
[1] “Early 2022 Heat Waves in Europe and climate change” by Happé, T., Vautard R., the students team of the June 2022 XAIDA Summer School and the XAIDA project participants.