POLICY BRIEF: Opportunities & Risks of AI-applications in Climate Sciences
This policy brief summarizes key learnings from the XAIDA and CLINT projects and formulates recommendations.
CLINT: CLimate INTelligence (GA N° 101003876) – Policy Brief
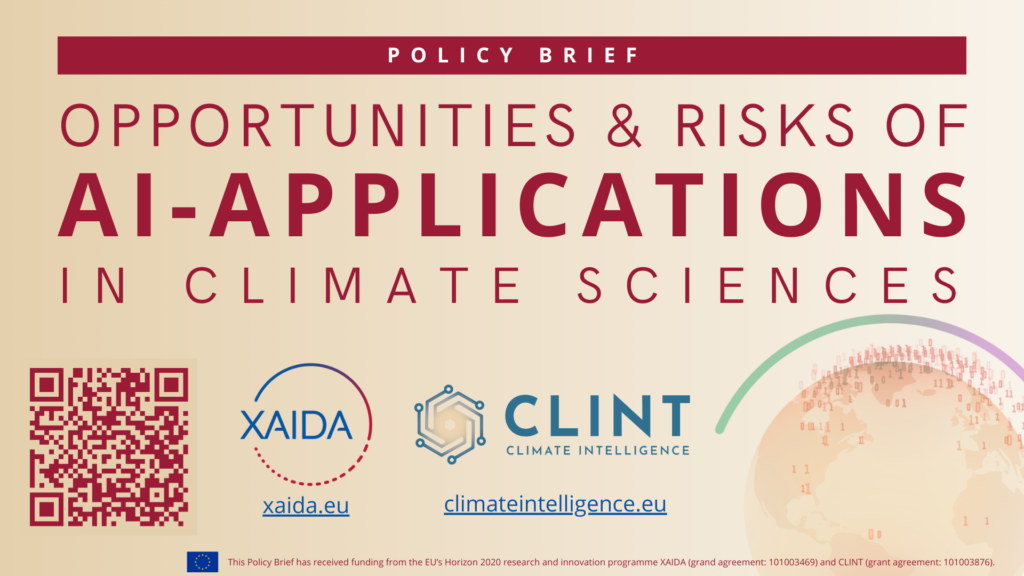
Artificial Intelligence (AI) is unlocking new frontiers in climate science and climate services—from improving impact-based seasonal forecasts to longer-horizon climate risk projections. However, the rapid growth of AI also brings challenges related to reliability, interpretability and trustworthiness. To fully harness AI’s potential while maintaining public trust, we need to invest in data-driven and hybrid models, transparent and open data practices, and robust validation. Funders, academics, and climate-tech startups must work together to develop scientifically credible AI-based climate services – at a time when informed responses to climate risks are more critical than ever.
The Horizon-2020 projects XAIDA (eXtreme events: Artificial Intelligence for Detection and Attribution) and CLINT (CLImate INTelligence) are funded under the same call and bring together European research institutes and climate risk practitioners to advance our understanding of—and response to—climate extremes. These initiatives highlight how Artificial Intelligence (AI) can unlock novel ways to detect, attribute, and predict extreme events and their impacts on multiple sectors. This policy brief summarizes key learnings from XAIDA and CLINT on using AI for climate research and climate services, addressing both the promises and the pitfalls, and provides targeted recommendations for policymakers, practitioners, and researchers.
In recent years, AI has made a remarkable entrance into climate research, revolutionizing weather forecasting. A major advancement has been the development of so-called ‘Foundation Models,’ which train Deep Learning (DL) architectures on large climate datasets to learn the complex underlying dynamics. Big tech companies like Google, Huawei, Nvidia, but also the European Centre for Medium-Range Weather Forecasts (ECMWF) have developed purely data-driven weather forecasting models that learn patterns directly from historical data. These AI models outperform traditional Numerical Weather Prediction (NWP) models in standard forecast verification scores for some meteorological variables and timescales.
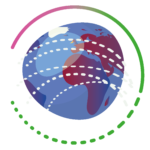
Science Message
Opportunity: AI and DL, including Foundation Models, have shown strong potential for short-term prediction and detection of extremes. While still in the early stages, longer-term forecasting (from seasonal to decadal and centennial scales) presents a major opportunity for DL. It has the potential to enhance predictive skill and uncover new sources of predictability, particularly when combined with explainable AI (XAI) methods. Additionally, AI methods can efficiently integrate impact data, enabling the development of actionable, impact-based forecasts that support better climate adaptation and risk management. Long-lead, impact-based forecasts would be of enormous societal benefit, providing a cost-efficient way to adapt to the increasing number and intensity of extreme weather events.
Challenge: Current DL methods often lack physical constraints, creating challenges in process understanding and attributing long-term trends or extreme weather events. Moreover, AI methods trained on present-day climate data may fail to generalize to future or past climates, complicating the creation of reliable counterfactual simulations for attribution. While DL applications remain difficult to interpret, their strong performance across diverse tasks highlights gaps in understanding why they work so well. This deserves deeper investigation.
Recommendation: Promote AI-enhanced forecasts that integrate meteorological data and impact data. Promote hybrid models combining AI and physics, enhance explainable AI, and integrate causal reasoning into DL models. Promote long-term collaborations between climate scientists and AI experts.
Climate services message
Opportunity: AI offers transformative opportunities for climate services, especially impact-based forecasts and climate risk assessments. AI enables us to tailor climate services products to the specific needs of clients in different sectors, including the water, energy, agriculture, and finance sectors. AI also enables the democratization of climate services via low-cost, local AI tools.
Challenge: The rapid proliferation of AI-driven tools in the climate-tech sector presents significant risks when deployed without a solid foundation in climate science. Many emerging startups market AI-based solutions with limited validation, transparency, or awareness of their scientific limitations. Without rigorous evaluation and adherence to established climate modeling principles, these tools may produce misleading climate risk assessments, potentially leading to poor decision-making, misallocating resources, and an erosion of trust in climate services. Ensuring scientific robustness, interpretability, and responsible deployment is essential to maintain credibility and maximize the benefits of AI in climate applications.
Recommendation: Promote the development and adoption of open-source AI tools, standardized methodologies, and best practices to ensure the responsible and transparent use of AI in climate science. Establish clear verification frameworks to enhance the reliability of AI-driven climate services and align them with EU regulations (e.g. EU’s Green Deal guidelines, EU’s Digital Services Act). Encourage stronger collaborations between climate-tech startups and academic institutions to bridge the gap between AI innovation and climate science expertise.
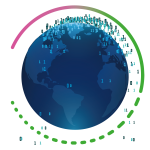
Glossary
Artificial Intelligence (AI): The ability of computer systems to perform tasks that typically require human intelligence, such as learning, reasoning, or problem-solving.
Deep Learning (DL): A subset of AI that uses large neural networks to automatically learn patterns from large amounts of data.
European Centre for Medium-Range Weather Forecasts (ECMWF): An independent intergovernmental organization, composed of national meteorological services as members, that provides global weather forecasts and develops state-of-the-art weather prediction models.
Numerical Weather Prediction (NWP) models: Traditional approach to forecast weather using computer-based models that simulate the atmosphere by solving physical equations.
Explainable AI (XAI): Methods and techniques in AI that make the decision-making process of models transparent and understandable to humans.
Hybrid models: Models that combine physical (e.g., physics-based NWP models) and data-driven (e.g., AI-based) approaches to improve performance and reliability of weather forecasts and climate predictions.
Authors
Dim Coumou, VU Amsterdam, Netherlands
Andrea Castelletti, Politecnico di Milano, Italy
Enrico Scoccimarro, CMCC Foundation, Italy
Gustau Camps-Valls, Universitat de València, Spain
Jakob Runge, Potsdam University and TU Dresden, Germany
Oana-Iuliana Popescu, Potsdam University and TU Dresden, Germany
Matteo Giuliani, Politecnico di Milano, Italy
Ilias Pechlivanidis, SMHI, Sweden
Schalk Jan Van Andel, IHE Delft Institute for Water Education, Netherlands
Nils Hempelmann, OGC/ECMWF, UK-Italy-Germany
Pascal Yiou, Institut Pierre-Simon Laplace, France