GENERATIVE NETWORKS FOR SPATIO-TEMPORAL GAP FILLING OF SENTINEL-2 REFLECTANCES
The paper is led by Maria Gonzalez-Calabuig (Image Processing Laboratory, Universitat de València).
Reference: Gonzalez-Calabuig M., Fernández-Torres, M.-Á. and Camps-Valls, G. (2025) « Generative networks for spatio-temporal gap filling of Sentinel-2 reflectances », ISPRS Journal of Photogrammetry and Remote Sensing, vol 220, pp. 637-648. https://doi.org/10.1016/j.isprsjprs.2025.01.016
Project Context:
Developed under the XAIDA initiative for extreme event detection & attribution with Artificial Intelligence.
Introducing GANFilling:
A GAN-based model leveraging ConvLSTM to fill VNIR gaps caused by various noise sources (clouds, shadows, missing data).
Key Innovations:
A new evaluation framework tailored for geoscience, adapting metrics like Kernel Inception Distance (KID) to handle both noisy and noise-free data.
Impact:
Improves kNDVI forecasting by ~70% across numerous European regions.
Abstract:
Earth observation from satellite sensors offers the possibility to monitor natural ecosystems by deriving spatially explicit and temporally resolved biogeophysical parameters. Optical remote sensing, however, suffers from missing data mainly due to the presence of clouds, sensor malfunctioning, and atmospheric conditions. This study proposes a novel deep learning architecture to address gap filling of satellite reflectances, more precisely the visible and near-infrared bands, and illustrates its performance at high-resolution Sentinel-2 data.
We introduce GANFilling, a generative adversarial network capable of sequence-to-sequence translation, which comprises convolutional long short-term memory layers to effectively exploit complete dependencies in space–time series data. We focus on Europe and evaluate the method’s performance quantitatively (through distortion and perceptual metrics) and qualitatively (via visual inspection and visual quality metrics). Quantitatively, our model offers the best trade-off between denoising corrupted data and preserving noise-free information, underscoring the importance of considering multiple metrics jointly when assessing gap filling tasks. Qualitatively, it successfully deals with various noise sources, such as clouds and missing data, constituting a robust solution to multiple scenarios and settings.
We also illustrate and quantify the quality of the generated product in the relevant downstream application of vegetation greenness forecasting, where using GANFilling enhances forecasting in approximately 70% of the considered regions in Europe.
This research contributes to underlining the utility of deep learning for Earth observation data, which allows for improved spatially and temporally resolved monitoring of the Earth surface.
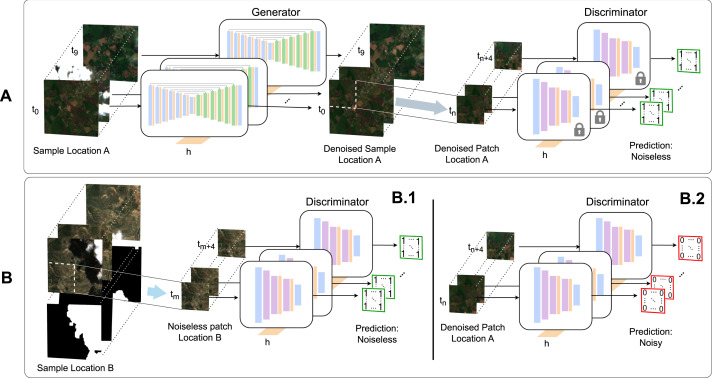