XAIDA WEBINAR | Ensemble Random Forest for Tropical Cyclone Tracking
XAIDA is now hosting an open monthly webinar. Within the XAIDA project, seventeen research institutes and climate risk practitioners, aim to develop and apply novel artificial intelligence methods to better assess and predict the influence of climate change on extreme weather. Join the webinar each month to dive into interesting topics such as machine learning for climate extremes, the societal impact of extremes, and education about climate change.
Coordination: Manon Rousselle (IPSL)
June 10th, 2025 at 10 AM (10:00) CET
Speaker: Pradeebane VAITTINADA AYAR, Postdoc at the Laboratory of Climate and Environmental Sciences (LSCE), Insitut Pierre-Simon Laplace (IPSL), CNRS
Title: Ensemble Random Forest for Tropical Cyclone Tracking
Abstract: « Even though tropical cyclones (TCs) are well documented during the intense part of their lifecycle until they start to evanesce, many physical and statistical properties governing them are not well captured by gridded reanalysis or simulated by Earth system models. Thus, the tracking of TCs remains a matter of interest for the investigation of observed and simulated tropical cyclones. Two types of cyclone tracking schemes are available. On the one hand, some trackers rely on physical and dynamical properties of the TCs and user-prescribed thresholds, which make them rigid. They need numerous variables that are not always available in the models.
On the other hand, trackers are leaning on deep learning, which, by nature, needs large amounts of data and computing power. Besides, given the number of physical variables needed for the tracking, they can be prone to overfitting, which hinders their transferability to climate models. In this study, the ability of a Random Forest (RF) approach to track TCs with a limited number of aggregated variables is explored. Hence, the tracking is considered a binary supervised classification problem of TC-free (zero) and TC (one) situations. Our analysis focuses on the Eastern North Pacific and North Atlantic basins, for which, respectively, 514 and 431 observed tropical cyclone track records are available from the IBTrACS database during the 1980-2021 period. For each 6-hourly time step, RF associates TC occurrence or absence (1 or 0) to atmospheric situations described by predictors extracted from the ERA5 reanalysis. Then, situations with TC occurrences are joined to reconstruct TC trajectories. Results show the ability and performance of this method for tracking tropical cyclones over both basins, and good temporal and spatial generalisation as well. RF has a similar TC detection rate as trackers based on TCs’ properties and significantly lower false alarm rate. RF allows us to detect TC situations for a range of predictor combinations, which brings more flexibility than threshold-based trackers. Last but not least, this study shed light on the most relevant variables for detecting tropical cyclones. »
Registration: xaidaproject@gmail.com
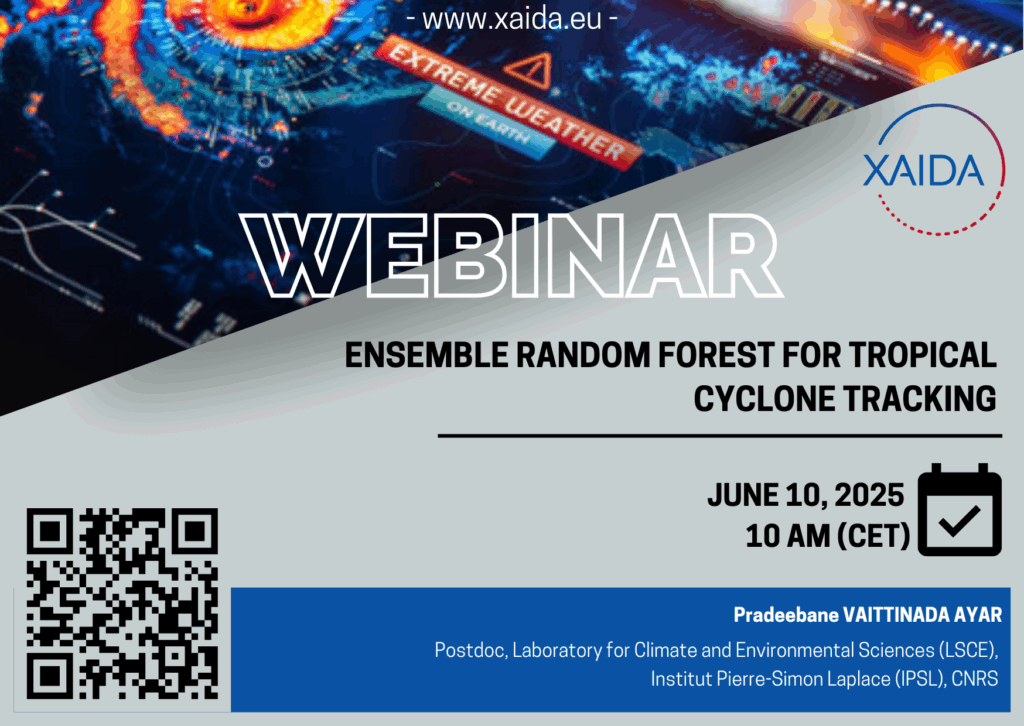